Educational influencers on Twitter. Analysis of hashtags and relationship structure
Abstract
In this article we research Spanish educational influencers with major presence on Twitter: what are the most common topics or hashtags used by them, whether there are groups based on the topics of their interventions or what type of social network they configure. To meet these goals, we selected 54 educational influencers with a high number of followers. We analyzed and classified the "hashtags" included in a total of 106,130 tweets. The analysis of hashtags has shown us that the most labelled topics correspond to educational content in different areas of the curriculum, collaboration, exchange and dissemination of digital materials, documents or resources, as well as activities related to training or discussion about innovative teaching methodologies. Using the Gephi software, we carried out a Social Network Analysis, determining the degree of centrality and betweenness centrality of the 54 influencers, which allowed us to identify influencers with greater recognition by the rest. Through a modularity analysis, we were able to identify five groups of influencers that do not work as closed groups but maintain frequent interactions with other influencers in other groups. This research highlights the need to better understand the contents and procedures that may promote informal learning by teachers.
Keywords
Digital activism, social network analysis, informal learning, teacher training, influencer, Twitter
Palabras clave
Activismo digital, análisis de redes sociales, aprendizaje informal, formación del profesorado, influencer, Twitter
Resumen
En este artículo indagamos acerca de los influencers educativos españoles con mayor presencia en Twitter: cuáles son los temas o hashtags más difundidos por ellos, las temáticas de sus intervenciones o qué tipo de red social configuran. Para dar respuesta a estos objetivos, en primer lugar, seleccionamos 54 docentes con alto nivel de seguidores. Analizamos y clasificamos los «hashtags» incluidos en un total de 106.130 tuits. El análisis de los hashtags nos ha mostrado que los temas más etiquetados corresponden a contenidos educativos en diferentes áreas del currículum, la colaboración, el intercambio y la difusión de materiales, documentos o recursos digitales, así como de acciones de formación o de debate sobre metodologías docentes innovadoras. Utilizando el programa Gephi hemos realizado un análisis de redes sociales, determinando el grado de centralidad y centralidad de intermediación de los 54 docentes, lo que nos ha permitido identificar influencers con mayor reconocimiento por parte del resto. A través del análisis de modularidad, hemos podido identificar cinco grupos de influencers que no funcionan como grupos cerrados, sino que mantienen frecuentes interacciones con el resto de influencers de otros grupos. A través de este estudio se pone de manifiesto la necesidad de conocer mejor los contenidos y procedimientos que pueden estar favoreciendo aprendizajes informales por parte de los docentes.
Keywords
Digital activism, social network analysis, informal learning, teacher training, influencer, Twitter
Palabras clave
Activismo digital, análisis de redes sociales, aprendizaje informal, formación del profesorado, influencer, Twitter
Introduction
The mechanisms that teachers use to improve their knowledge and skills have changed radically in recent years. The emergence of social networks such as Facebook, Twitter or Instagram has allowed teachers to choose what they want to learn and who they trust to guide their learning. As we move forward in the digitization of society, there seems to be a growing consensus on the need to adopt a broad perspective on activities that promote teachers' professional development. Currently, such development has been considered as the “coherent sum of activities aimed at improving and expanding teachers’ knowledge, skills and conceptions so that they can assume changes in their behavior and way of thinking” (De-Rijdt et al., 2013: 49). Thus, professional development is not limited to formal training scenarios, but includes a broader and more varied set of non-formal and informal training activities (Bound, 2011; Russ et al., 2016; Moore & Klein, 2020).
One of the ways in which many teachers keep up to date involves the use of social networks. Recent studies have focused on analyzing how and why teachers use social networks for professional development, and as spaces to establish contact with other teachers, creating opportunities for affinity and collaboration (Carpenter et al., 2020). Social networks allow us to expand what has been called social capital (Rehm & Notten, 2016). Through them, they generate interactions that can be stable or temporary, allowing teachers to gather resources or obtain information from other people considered relevant to their work (Fox & Wilson, 2015).
Social networks create social capital not only through the exchange and dissemination of information. The social capital they generate can also be analyzed through the influence, control and power that can be granted to those with whom an informal monitoring relationship is established (Adler & Kwon, 2002; Seok-Woo & Adler, 2014). To understand the process by which social networks generate social capital, Nahapiet and Ghosha (1998) established three elements that must be taken into account: structure, relationships and cognition. The structure refers to the patterns that configure the network, its morphology, density and internal hierarchies among members. The relational dimension is associated with the type of interactions that take place in the network. Finally, the cognitive dimension refers to the contents, resources, interpretations and meaning systems shared by the members of a network.
Following Nahapiet and Ghosha (1998), one characteristic of the network structure is related to leadership. In every network there are people who play a prominent role and are considered opinion leaders. Opinion leaders (influential teachers) are those people who occupy a central and structural role in a network (Rogers, 2005). They are people who can influence the attitudes or behavior of other people consistently over time (Del-Fresno García et al., 2016). They exercise this role to the extent that they are accepted by others (peripheral actors) as influential subjects (Wang & Fikis, 2017). As demonstrated by Izquierdo-Iranzo and Gallardo-Echenique (2020) when studying the “studygrammer” phenomenon, these subjects can be adults or not, namely young people who create informal learning opportunities for their peers on Instagram (Izquierdo-Iranzo and Gallardo-Echenique, 2020). Another component identified by Nahapiet and Ghosha (1998) in relation to the social capital generated by social networks is cognition. Analyzing it within social networks, we can refer to hashtags or labels that serve to group certain topics present in a Twitter publication. A hashtag gathers words or groups of words preceded by the # symbol that allows users to participate in conversations on a specific topic and thus group them under the same label. They also serve to organize and structure these conversations, making it easier for users to find tweets on a particular topic or discussion (Greenhalgh & Koehler, 2017). They can be understood as "affinity spaces" (Gee, 2005) in that certain users find others akin to their interests based on the tags they use (Rosenberg et al. 2016). According to Gee (2005: 67), an affinity space is a "place where people affiliate with others based primarily on shared activities, interests, and goals". These spaces generate communities comprised of people who seek connection and collaboration with each other (Carpenter et al., 2020). Affinity spaces encourage users of the same social network to meet and interact around a common interest, hobby, identity or ideology. The environments of these affinity spaces can be varied. In their study on the use of social networks among young people, García-Martín and García-Sánchez (2015) determined that the main reason for using these networks, including Twitter, was to have fun or be entertained. Similarly, in another analysis developed on the patterns of use of social networks and web 2.0 environments among young Spaniards, platforms such as Twitter or YouTube were found to provide greater personal satisfaction among young people (García-Martín & García-Sánchez, 2015).
Affinity spaces can create the conditions for teachers' informal learning. Eraut (2004) differentiated three levels of intention in informal learning: implicit learning, reactive learning and deliberative learning. Deliberative learning is the one that interests us in this paper, since it occurs when teachers intentionally seek and receive information through digital media, coming from opinion leaders (influencers) whom they trust and follow (Van-Den-Bossche & Segers, 2013). In the case of Twitter, Santoveña-Casal and Bernal Bravo (2019) showed how the use of this network improved the motivation and satisfaction of teachers in training. Other analyses carried out on Twitter have made it possible to investigate some of the topics or hashtags that are most frequently used in the educational and academic sector (Carpenter et al., 2020). We are referring about hashtags such as #michEd (Greenhalgh et al., 2016), #Edchat (Britt & Paulus, 2016; Staudt-Willet, 2019) and #PhDChat (Veletsianos, 2017).
In this article we focus on Twitter as the main affinity space. Following the dimensions identified by Nahapiet and Ghosha (1998), we set out to answer the following questions: Who are the most prominent Spanish educational influencers on Twitter? What are the most popular topics or hashtags among Spanish educational influencers? What is the relational structure that characterizes the network of Spanish influencers on Twitter? In this article we use the term "influencer" to refer to those educators who have an active presence in social networks. We understand that the term influencer may not be accepted by some of the subjects investigated due to the possible commercial or merely recreational connotation of the activity.
Material and methods
In this study we aim to understand the relational structure and content promoted by Spanish educational influencers on Twitter. Twitter is not the only social network. Other studies have analyzed how teachers use Facebook (Hart & Steinbrecher, 2011) or Instagram (Carpenter et al., 2020). We chose this social network because, as indicated by Luo et al. (2020) in their recent review, it is the main platform for creating professional learning networks and sharing knowledge. The first objective was to identify those people who could be classified as "educational influencers" with relevance on Twitter. To do this, we used the Buzzsumo software, a marketing analytics tool that enables advanced queries to be performed on people, profiles and topics most consumed and shared on social networks. We started by filtering users by country (Spain) and keywords present both in the user's biography and in their publications. The keywords used for this ranking were: enseñanza [teaching]; soyprofe [iamateacher]; educación [education]; escuela [school]; profesor [teacher]; aprendizaje [learning]. From this analysis we obtained a sample of 64 Spanish profiles from which we initially selected the ten most representative. We considered as outstanding influencers those with more than 15,000 followers and more than 5,000 tweets published. The profiles initially selected were the following: @xarxatic; @ScientiaJMLN; @Manu_Velasco; @maestradepueblo; @unicoos; @smoll73; @salvaroj; @ftsaez; @edusadeci; @AyudaMaestros.
Once the most prominent Spanish influencers had been identified, we proceeded to analyze the mentions that made by them in their tweets. We were interested in finding the people whom these teachers considered relevant, and who were included in their mentions. The analysis of mentions showed different types of profiles on Twitter: individuals, institutions (government, agencies, official centers), politicians (ministers), national and educational press, radio, television, music or universities. We selected only those mentions that referred to individuals. Once the list of subjects mentioned by these ten influencers was configured, we established as a criterion that they were mentioned by at least four of the influencers in our sample. This process allowed us to identify a total of 44 new influencers, which, together with the ten initial influencers, make up the sample of 54 subjects analyzed.
The list of influencers is as follows: @aaronasenciofer; @AgoraAbierta; @anam_cid; @anatorres8; @AyudaMaestros; @bpalop; @bvicario2013; @c_magro; @carmeniglesiasb; @cccesssarrr; @ClaraGrima; @cpoyatos; @davidcpvm; @DechantCarla; @doloresojeda1; @edusadeci; @eliatron; @Estebandelashg; @ftsaez; @garbinelarralde; @Gorkaprofe; @history_topics; @hruizmartin; @imgende; @javierfpanadero; @JavierPalazon; @jblasgarcia; @jhergony; @jordi_a; @JorgeRuizMN; @Lamunix; @lepetitpan; @londones; @LUISDAVILABAND1; @maestradepueblo; @Manu___Velasco; @manueljesusF; @manuparadas; @MiriamLeiros; @monparaiso; @Nando_Lopez_; @octavio_pr; @OscarRecioColl; @peralias; @PsicEduM; @ramon_besonias; @raulillodiego; @salvaroj; @ScientiaJMLN; @smoll73; @tonigarias; @tonisolano; @unicoos; @xarxatic.
Of the 54 Spanish teachers selected, 38 are male and 16 are female. With respect to the educational level towards which their interventions are oriented, we found 23 users whose publications are mainly directed towards primary education, 10 towards secondary education and high school, 21 focus their tweets on higher and university education and four of them also address conversations and content related to early childhood education.
To address the second research question, we focused on the hashtags used by the selected influencers. To obtain the data, we used, throughout the month of September 2020, the Twlets application, which is an application that enables the download of a maximum of 3,200 tweets from each subject in Excel format. We collected 106,130 hashtags included in a total of 167,162 tweets from the 54 selected influencers.
In relation to the third question, we analyzed the structural and relational dimensions of the influencers' network through a process of social network analysis (SNA). To do this, we analyzed the mentions made by each of the 54 selected subjects in their Twitter messages about the rest of the influencers. To carry out this study we used data obtained from 167,162 tweets from the 54 profiles. Once this data was downloaded, we proceeded to extract the mentions. This compilation of frequencies generated an adjacency matrix that shows the number of times each influencer mentioned and was mentioned by each of the other 53. It should be noted that in this case we found some self-mentions by some of them. Once the adjacency matrix was created, we used the program GEPHI 0.9.2 (Bastian et al., 2009) to analyze the relationships. Gephi is an open-source program that allows interactive visualization of networks and provides the tools to generate dynamic and hierarchical graphs.
Results
# Hashtag content analysis
One of the aims of this article was to inquire about the affinity spaces that, according to Gee (2005), allow teachers to create shared activities, interests and objectives. To this end, we analyzed the hashtags that the selected influencers included in their messages. Of the total of 106,130 hashtags, we grouped them into 22,812 and classified them according to their content. The criterion for including a hashtag was to have more than 30 mentions. Table 1 shows the categories and subcategories found in the analysis, indicating the frequency in the total number of subjects.
First, we found a set of hashtags that we have called Generic and that refer to general educational concepts, such as #educación [education], #profesores [teachers], #aprendizaje [learning], #tic [ICT] or #redessociales [socialnetworks]. A second set of hashtags that we want to highlight includes references to processes of collaboration, exchange, support and, in short, informal teacher learning. The results show us that through these hashtags teachers assume a commitment to share and support other teachers. Of all the hashtags used in this category, we must highlight the one called #claustrovirtual [virtualfaculty], which has a frequency of 1058 references. This hashtag is particularly relevant because, although it was created before the pandemic, it has proved to be a means of support for teachers who, through it, shared their doubts, concerns and questions about tools, resources and materials for online teaching. On the other hand, Twitter has also been used by educational influencers as a space to disseminate more or less formal training initiatives: conferences, colloquiums, seminars, MOOCs, organized by institutions or by individuals.
A fourth category found in the analysis of hashtags is what we call educational content. These are links to addresses, resources or pages related to: History, Reading, Mathematics, Science, Language and Literature, Drawing, Music, Physics, Art, Physical Education, Philosophy or Drawing. Along with educational content we find hashtags that refer to teaching methodologies that could be characterized as innovative: visual thinking, gamification, problem-based and game-based learning, flipped classroom, etc. Special attention should be paid to hashtags related to the coronavirus. Obviously, this topic has occupied an important part of the messages by the educational influencers analyzed.
The hashtags we have analyzed show content that is closely related to the training needs identified by the TALIS report (OECD, 2014). This report highlighted that the main need expressed by teachers was to improve their knowledge of the content they teach, as well as the way they teach it. In this sense, the existence of hashtags related to innovative methodologies shows the interest not only of influencers, but of followers in general to inquire about new ways of teaching. The same is true for attention to diversity and inclusion. On the other hand, the numerous hashtags that emphasize support and collaboration among teachers (#recomiendo [irecommend], #profesesinnovadores [innovatingteachers], #sosdigitaldocente [sosdigitalteacher], #compartirentiemposdecoronavirus [sharingintimesofcoronavirus], #profesesqueayudan [teacherswhohelp], etc.) show us a trend towards a horizontal relationship, of selfless support among the teachers participating in the network.
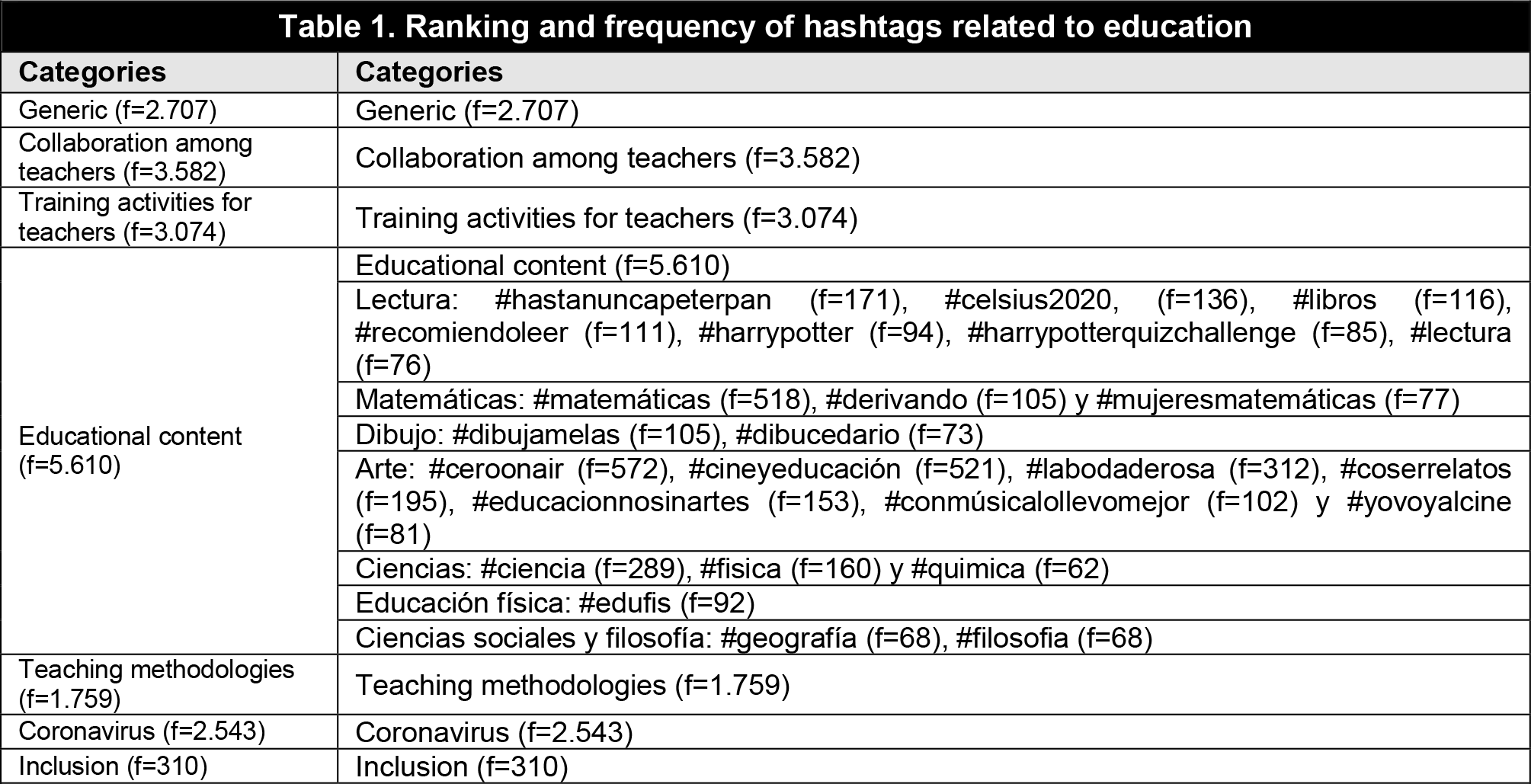
Analysis of structure and relationships in the influencer social network
The hashtag analysis previously carried out provides an initial idea of the number and content of the interactions of the 54 subjects in our study. But, in order to know a little more about the structure and relationships between these educational influencers, we also analyzed the network of interactions they generate. The network we analyzed is an undirected network, since each member can mention and be mentioned by any other member. The analysis with the Gephi tool allowed us to create a network of 54 nodes (subjects) and 1,607 edges (relationships between nodes). One aspect of interest when analyzing a social network has to do with determining which members (nodes) of the network play an important role both in terms of the level of connections they have with the rest of the members and the quality of these connections. One of the parameters usually analyzed in a network is its density (the ratio between possible and actual connections) (McCulloh et al., 2013). A network will be very dense when the number of relationships between its members is very high, close to the maximum possible. In the case of the influencers' network we are analyzing, its density is average, i.e. it does not stand out either for the intensity of relationships or for the lack of them.
Together with density, it is important to analyze the concept of centrality in the network, since it enables the identification of the most prominent nodes in the network (Del-Fresno et al., 2016). Centrality can be analyzed using Gephi network analysis in two main ways: degree centrality and betweenness centrality. The degree of centrality of a person in the network has to do with the number of relationships he/she maintains with the other members. The relationships can be in-degree (in our case, the number of mentions that the other people in the network make of the influencer in question) or out-degree (the mentions that the subject makes of the other network participants).
Table 2 shows these data for those influencers who obtained a grade higher than 70. We can see that in most cases the input grade is very similar to the output grade. This means that teachers mention as much as they are mentioned, which represents a balanced flow in the communication process within the network.
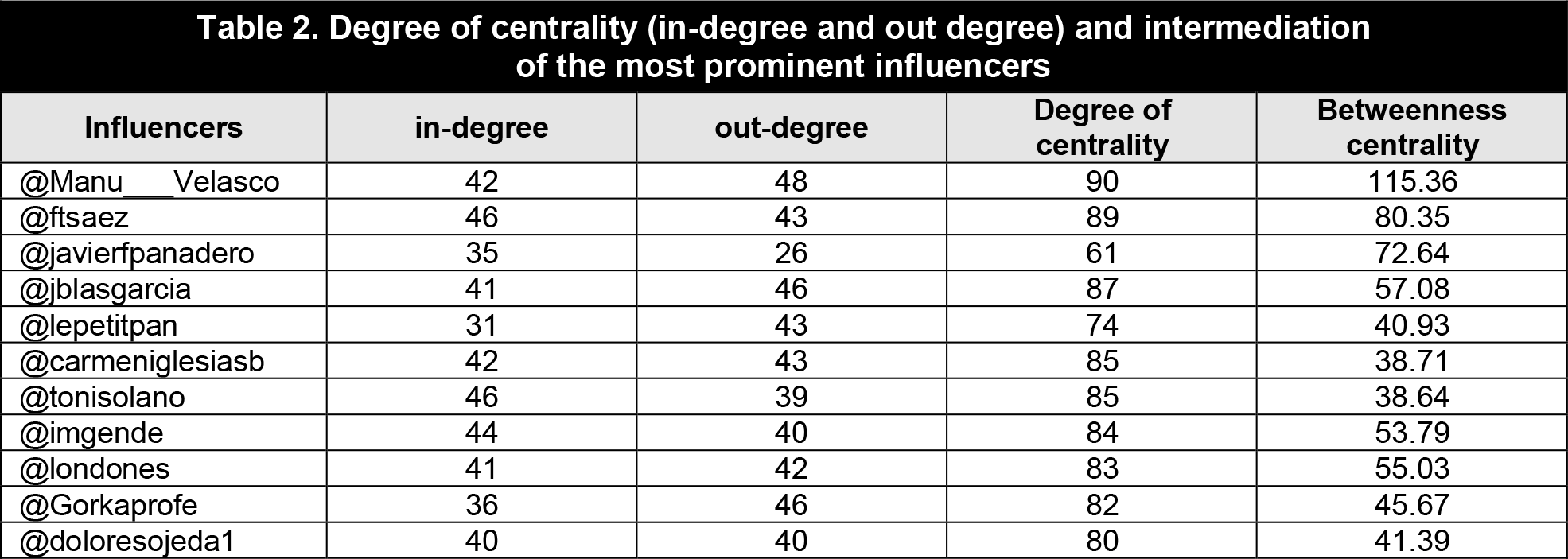
The degree of centrality of an influencer is an important indicator of his or her level of influence in the network. But along with this index, network analysis incorporates another index called betweeness centrality. In this case, it is not only a question of knowing the centrality of a subject, but the extent to which he/she is a necessary intermediary for information to flow to another subject. Betweeness centrality is important to determine how a person in the network is key for information to flow (or not) to other subjects or nodes. In this case, we find influencers who stand out in the degree of centrality and at the same time in the betweeness centrality, and others who stand out in only one of these indexes.
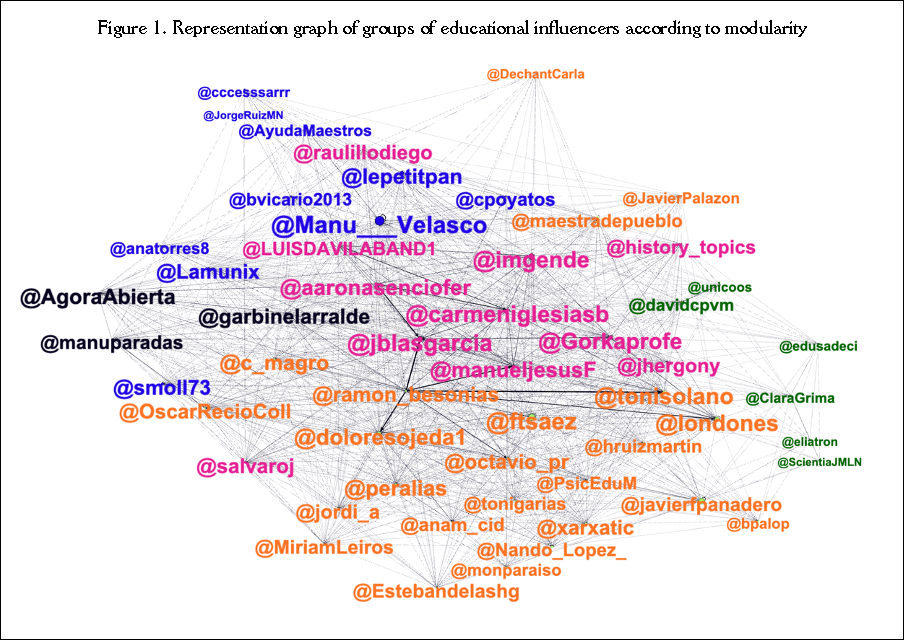
But beyond the identification of these apparent individual leaderships, we were interested in finding out whether there are specific synergies between certain influencers. To this end, we have applied the modularity analysis offered by Gephi. Modularity is a measure that establishes the structure of a network, in order to identify groups or clusters of subjects or network nodes. When modularity is high, the groups are homogeneous among themselves and with little interaction with the rest of the groups. When it is low, there are frequent interactions between the different groups (Sihag et al., 2014). The result of the modularity calculation of the network we are analyzing is low: 0.261. This means, as we have indicated earlier, that it is a group of people who interact openly with other individuals. But the modularity analysis also allows us to create groups of influencers who share a special affinity, and for this purpose Gephi uses the Louvain algorithm (Blondel et al., 2008). This algorithm allows the creation of communities of individuals sharing affinity spaces (Gee, 2005). The result of the modularity analysis allowed the identification of five groups of influencers.
As can be seen in Figure 1, the different colors identify the subjects that are grouped through modularity analysis. We can observe that the size of the label of each node is different because it represents the degree of centrality of each one. To the extent that the text is larger, these are teachers who mention and are mentioned in high frequency. The first group, (green color) is comprised of six members: @davidcpvm, @ClaraGrima, @edusadeci, @unicoos, @eliatron and @ScientiaJMLN. This group includes subjects whose common ground is the teaching of science and mathematics at different educational levels. We find the user @davidpvm, the founder of the YouTube channel @unicoos, and other teachers dedicated to mathemathics teaching, such as @ClaraGrima, @edusadeci or @eliatron.
In the second group (blue) we find a clear affiliation of these influencers in terms of the dissemination of educational information or knowledge in the press or magazines. They are subjects who usually participate as authors or contributors in educational magazines and/or the press. We find examples such as @Manu__Velasco, collaborator in @AyudaMaestros, teacher and writer. Similarly, @Lamunix, vice president of Espiral Association, Education and Technology. They usually write about innovation in teaching and teacher training (especially in methodologies and technologies for teaching), as in the case of @cccesssarrr, communicator and teacher who collaborates in articles and publications published by Fundación Telefónica. The last and clear example is @anatorres8, journalist at @el_pais newspaper and writer on educational topics.
The third group of influencers is the largest (orange), comprising 24 people. Given the wide variety within this group, we performed a specific modularity analysis within the same group. We then obtained two subgroups that show significant similarities, allowing us to group these subjects. On the one hand, we found a group of current university teachers, such as @ftsaez, professor and communicator at the University of Granada, as well as @jordi_a, teacher of educational technology at Jaume I University. This group also includes @balop, professor in the area of Didactics of Mathematics at the University of Valladolid. We found university teachers, as well as teachers who are experts in continuous teacher training, such as @smoll73 or @JavierPalazon, author and coordinator of the virtual magazine Educación 3.0 and @c_magro, educational consultant in digital strategy. On the other hand, in the second subgroup, we observe a grouping of female teachers who teach at different levels of the compulsory education system. We find primary school teachers such as @doloresojeda1, a language and literature teacher and @MiriamLeiros, a social studies teacher and activist in TeachersForFuture, a digital platform that brings together teachers in the fight for climate change. This group also includes pedagogues and psychologists such as @anam_cid, who is a teacher and psychologist, and @peralias, pedagogue and retired teacher. This grouping clearly shows how the teachers who belong to the group have similar profiles not only in the contents they publish, but also in what they share with their followers.
In the fourth group (black) we find people whose profile is close to experts and/or communicators who are specialized in innovative and technology-based teaching methodologies. In this group we include @AgoraAbierta, a trainer specialized in gamification and Visual Thinking, or @garbinelarralde, a high school teacher of technical drawing and ICT coordinator, whose publications often mention the Visual Thinking methodology. In addition, we find @manuparada, a primary education teacher who mentions methodologies such as problem-based and game-based learning in his interactions.
Finally, the fifth group (pink) includes 11 influencers who interact regularly by sharing resources, materials and information they find on Twitter. They often post tweets intended to express acknowledgement or to share resources that reach them through different followers. These tweets are not directed to a single user but are rather focused on the mention of several subjects to disseminate the work or digital resources created by the teachers’ followers. They take on the task of disseminating and recognizing the work created by teachers, enabling them to reach more followers. As mentioned, we find profiles as diverse as that of @imgende, the person with the highest "indegree" rate in this group. This may be explained by the fact that she is one of the most active teachers in our social network analysis, being a university teacher who designs and disseminates resources and materials so that other teachers can acquire knowledge and strategies for their online teaching. We found other teachers who, altruistically, design and share materials with the rest of the community, such as @Gorkaprofe, a primary school teacher, in subjects such as robotics, mathematics, ICTs, etc. or @aaronasenciofer, a primary school teacher who regularly creates materials on cooperative learning, gamification and flipped classroom and shares them with his community.
Discussion and conclusion
Throughout this article we have made a first approach to find out who are the teachers who have a proactive presence on Twitter, their interaction with each other and the content of their messages. These influencers create what Gee (2005) calls "affinity spaces" around a wide variety of educational topics. By tagging their own messages or retweeting the messages they receive, these influencers somehow establish and legitimize certain topics with a variety of content (Wenger, 2000). The analysis of hashtags shows that the most tagged topics correspond to educational content in the different areas of the curriculum. This finding should not be surprising given that the content taught is one of the main concerns of teachers (Berry et al., 2016). The influencers studied act as "knowledge brokers" (Plair, 2008), or necessary intermediaries who select, create, share, evaluate resources or information. The lockdown resulting from the pandemic has led many teachers to create and share educational resources and make them available to other teachers. Some of these hashtags were ephemeral, but some have been maintained throughout this time, such as the case of #claustrovirtual. This is an example of how Twitter can shape a community of practice (Wenger, 2000) and be a space that creates "opportunities for social, situated and distributed learning among teachers" (Luo et al., 2020: 1675) as it contributes to blurring the boundaries between formal and informal teacher learning (Evans, 2019).
However, in addition to the educational content shared on the network, in this study we have investigated the structure and relationships that occur among the 54 influencers analyzed. Using a social network analysis (SNA) approach, we have identified, first of all, the subjects who have a high degree of centrality in the network. These are teachers who are recognized as influencers by a significant proportion of their peers. In addition to the degree of centrality, we have also identified influencers who play a key role in the circulation of information in the network studied. The social network analysis carried out has allowed us, through modularity analysis, to find five groups or clusters of influencers with different affinities. These groups do not have exclusive relationships with respect to influencers in other groups. Rather, they are groups that, while sharing some characteristics of their own, tend to interact with the rest of the groups. In some way, the influencers we have analyzed share common characteristics with activists in other fields. The analysis of recent social movements (Occupy Wall Street, Indignados and UK Uncut) conducted by Gerbaudo (2017) shows a common orientation in relation to three so-called "techno-libertarian" principles: transparency (tendency to openness, to open-source), horizontality (rejection of formal hierarchies) and denial of leadership (tendency to assume that leadership is something to be avoided).
Although in this article we did not analyze the extensive content of the messages, the analysis of the hashtags shows a clear coincidence with the above principles. Through the analysis of hashtags, there is a tendency to share resources or learning objects that are open and freely accessible (transparency). On the other hand, as we have analyzed, the network of 54 influencers is self-organized horizontally, with some groups having common characteristics but interacting with the rest of the groups, and where teachers of all educational levels are equally mixed.
Finally, the denial of leadership is something that we have found informally when interviewing some of these influencers as part of the research that we now present. As we can see, the influencers analyzed operate as opinion leaders to the extent that they not only redistribute information, but also generate their own information, either because they design content or because they find first-hand information based on their position in the network (Walter & Brüggemann, 2020). We can thus assume that digital social networks are enabling the establishment of new informal leadership among teachers (Carpenter & Harvey, 2019). Through them, opportunities are created so that, by sharing their experiences, ideas, conceptions and reflections, teachers can develop personal or professional learning (Greenhalgh et al., 2016). The results of this study show the need for a better understanding of how these informal leaderships are generated among the influencers analyzed, their perceptions, intentions, orientations and principles that lead these people to share their time, knowledge and resources with other people in the network. However, although this is important, it will also be necessary to understand the point of view of the recipients of these interventions. By understanding who follows these influencers, we will be able to determine whether the information distributed on the network is really transformed into knowledge and teaching practice. We should not assume that the information, resources and content shared directly generate learning in teachers. Research must determine what is learned, what is applied, how the transfer of learning occurs and what the results are for teachers' professional development.
Finally, this study has some limitations. First, the selection of the influencers was made on the basis of the number of followers. Also, additional criteria could have been considered for their selection. Secondly, the complete content of the messages has not been analyzed but only the hashtags, with the understanding that such tags summarized the content. Thirdly, an evolutionary study of the hashtags over time was not carried out. Knowing the evolution of certain hashtags over time could help us to know which are trending topics and which are permanent topics that concern teachers. (1)