COVID-19 on YouTube: Debates and polarisation in the digital sphere
Abstract
Social media has significantly transformed how political discussions and deliberations occur, mainly by providing a digital realm for the public sphere. This study aims to analyse the extent of polarised opinions across Spain, Italy and the United Kingdom regarding COVID-19 during 2020 within social media. To do this, we examined YouTube comments (n=111,808) using automatic analysis and machine-learning techniques based on algorithms. This methodological strategy denoted an innovative and unique quantitative approach for this field of study. In line with previous research, the hypothesis was that the degree of polarization does not crystallize in the same manner in different countries’ digital spheres. Therefore, it could be said that higher levels of polarization occur amongst Southern European countries like Spain and Italy (both countries adhering to a polarised pluralism model), compared to other countries ascribing to the liberal model (the United Kingdom in our study), which provides evidence supporting previous research studies. The results confirmed the hypothesis that the polarization of digital deliberation between Spain and Italy is higher than in the United Kingdom. But, also, the findings based on more disaggregated analysis suggest that the most polarized attitudes are even rewarded by other users in Mediterranean countries.
Keywords
Public sphere, YouTube, cyberpolitics, deep learning, polarisation, COVID-19
Palabras clave
Esfera pública, YouTube, ciberpolítica, aprendizaje profundo, polarización, COVID-19
Resumen
Las redes sociales han transformado de forma muy significativa la forma en la que se produce el diálogo político, impulsando una configuración digital de la esfera pública. El presente artículo tiene como objetivo el análisis de la deliberación producida en las redes sociales, con un especial énfasis en la polarización. Tomando como referencia los comentarios observados en YouTube sobre la COVID-19 durante 2020 en España, Italia y Reino Unido, lo cual arroja una muestra de 111.808 comentarios, se aplicaron una serie de técnicas automáticas de análisis basadas en algoritmos, lo que supone una metodología cuantitativa novedosa en este ámbito de estudio. En línea con lo señalado por trabajos previos, la hipótesis que se plantea en este artículo es que el grado de polarización no se da con la misma intensidad en las esferas digitales de distintos casos. De esta manera, cabe esperar unos mayores registros de polarización en la esfera digital de los países del sur de Europa, adscritos a un modelo de pluralismo polarizado, que en países de otros modelos como el liberal. Los resultados confirman la hipótesis, verificando que no solo se observa mayor polarización en España e Italia que en Reino Unido, sino que, a nivel desagregado, los hallazgos apuntan a que la actividad más polarizante obtiene mayor aprobación en los países mediterráneos de nuestra muestra.
Keywords
Public sphere, YouTube, cyberpolitics, deep learning, polarisation, COVID-19
Palabras clave
Esfera pública, YouTube, ciberpolítica, aprendizaje profundo, polarización, COVID-19
Introduction and state of the art
The emergence and rapid development of digital technologies has ushered in a paradigm shift that affects both the media ecosystem and the configuration of the public sphere (López-García, 2005; Arias-Maldonado, 2016). Theorists have defined this concept as the communicative space in which issues of general interest are considered for discussion by concerned/affected citizens, fulfilling a series of admittedly ambiguous, normative criteria (Dahlberg, 2004). This process requires the mutual understanding of the participants, as well as a genuine, honest effort to reach a consensus (Serrano-Contreras et al., 2020).
The model shift, already identified by Jay Blumler (2018), has been linked to the concept of crisis (Davis, 2019). The connection seems even more evident during the pandemic, and has had a substantial impact on the transformation of the public sphere. In this sense, the theorisation of public spaces raises a new analytical perspective based on the concept of public horizons (Volkmer, 2014), which highlights the centrality of media in a constant process of evolution, in which the particular prominence of digital media is confirmed. The development of processes involving extreme fragmentation of the contemporary public sphere, accelerated by the environment imposed by digital communication, has led to a new definition of transition, such as the ‘post-public sphere’ (Davis, 2019; Schlesinger, 2020; Sorice, 2020).
The implications of the definitive expansion of social media are enormous. The consolidation of different forums such as Facebook, Twitter or YouTube is a good example (Dougan & Smith, 2016). The logic underpinning the functioning of social networks has also transformed the way in which political phenomena are received, perceived and discussed in the public sphere (Fung et al., 2013), which has now become a digital realm. However, the literature does not take for granted the positive effect on the deepening of political systems, and we can observe opposing views and arguments about the nature, dynamics and profile of this alteration (Gozálvez-Pérez, 2011).
On the one hand, the digital world has been consistently emphasised as the hope for promoting civic engagement, offering a wide array of democratic innovations that help make political discourse more pluralistic, facilitating greater involvement in public affairs, enabling citizens to monitor and control power, producing more information and providing new formats for the transmission of political content, and eventually culminating in participation in decision-making. So-called cyber-optimism (Bruns, 2008) suggests that ICTs could serve to articulate a more relaxed conversation among citizens, which does not happen in highly formalised, deliberative forums, helping to increase their nodal nature (Margetts, 2009). Through conversation, members of society clarify their own views, learn about the opinions of other interlocutors, and determine what problems citizens are facing (Stromer-Galley & Wichowski, 2011). Indeed, talking about issues of general interest with other citizens is considered necessary for a comprehensive understanding of democratic coexistence and thus for giving meaning to participation in political life (Rubio, 2000; Scheufele, 2001). To the extent that interaction is a vital component of democratic societies, these processes could lead to more inclusive and meaningful public deliberation (Bimber, 1998; Berry et al., 2010). In this sense, social networks show great potential in mobilising and empowering citizens, and in facilitating options for interacting with each other (and with their representatives), completely outside the more institutionalised, mainstream communication channels in the hands of large media corporations.
On the other hand, a more critical group of sceptical scholars (Fuchs, 2017) has argued that, far from the aforementioned optimism, the observed dynamics point to the fragmentation of this digital sphere and the consolidation of filter bubbles and echo chambers, phenomena whose scrutiny poses a number of significant methodological challenges, and which we have only recently begun to understand (Pariser, 2011) 1 . To the extent that there are different social networks and, within these, an almost infinite variety of distinct compartments, regular users end up choosing their interlocutors. In this sense, networks constitute echo chambers where we only hear the echo of our own voice (Sunstein, 2008), such that social empathy can be seriously damaged by identifying a drastic suppression of exposure to diversity (Prior, 2007) and a clear ideological homogeneity (Valera-Orgaz, 2017). Likewise, the high level of anonymity hidden in the network could constitute a means to exacerbate uninhibited communicative behaviour, moving in the direction of an increase in ill-mannered, disrespectful, uncivil, or aggressive political discussion (Rowe, 2014). In this vein, rather than facilitate rational and informed deliberation, networks function by amplifying and modulating an atmosphere or public mood that is sometimes unreflective, manipulated and full of noise; this hinders calm reflection, underlining the notion of affective resonance (Fleig & von-Scheve, 2020). As Sunstein (2008) points out, given that the fragmentation of public opinion can reduce social cohesion, networks can make contact between different opinion groups more difficult, thus deepening the radicalisation of one’s own opinions by never confronting their opposites (Reese et al., 2007).
At the same time, and perhaps related to the above, polarisation, or affective polarisation (Iyengar et al., 2019), has been incorporated as one of the main features of social and political phenomena in recent years, becoming an extremely important object of study in the field of political communication. Polarisation can be defined as the relative distance between two opposing political viewpoints. However, it is a concept in constant readjustment, although its primary meaning has to do with the growth of the space between poles, which is caused primarily by the influence of emotions and beliefs, rather than by reason and evidence (Mason, 2014; Olsson, 2013). These distinctions can lead to extreme positions (Fletcher & Jenkins, 2019; Gidron et al., 2019).
The aim of this proposal lies in the study of political deliberation from the angle of polarisation, which will be traced in a comparative way in relation to COVID-19, an issue that could be qualified as commonplace, as it is situated in the field of public health. Specifically, this article will explore the level of polarisation observed on the YouTube network during 2020 in relation to this matter in Spain, Italy and the UK, countries that represent differentiated models in political communication studies (Hallin & Mancini, 2004). To this end, the most relevant comments in connection to this episode will be examined using automatic analysis techniques based on algorithms, which is a novel, quantitative method in the field of study involved. Most of the research on political deliberation in recent years has focused on interactions observed on Twitter and Facebook (Bakshy et al., 2015; Conover et al., 2011; Gruzd & Roy, 2014; Jaidka et al., 2019; Oz et al., 2018). Underlying this is not only the projection of traditional leadership, but also a relatively simple collection of data in practical terms through its API. YouTube, on the other hand, has transitioned from a repository platform for audiovisual material to an environment that could be considered a social network several times the size of Twitter (with over 2 billion users versus Twitter’s 340 million), and which offers similar interaction features with the inclusion of a recommendation system, the "likes", and data management (Allgaier, 2019).
Materials and methods
The hypothesis was formulated based on the following conditions: in countries with polarised pluralism models (Spain and Italy, in our case), a greater polarisation can be expected when analysing the political deliberation produced in the digital sphere compared to countries founded on the liberal model (United Kingdom). This hypothesis is supported by the differences revealed between these two systems where, moreover, in recent years, polarisation has been challenged by the profound transformations experienced due to both the unexpected electoral outcomes in some countries, and the impact of certain issues that abruptly burst onto the scene of public opinion (e.g. the Brexit referendum). If positive, it would confirm findings that affective polarisation is not the same in all countries (Boxell et al., 2020; Fletcher et al., 2020). In other words, polarisation may have a dependency relationship with regard to the society being examined, and not necessarily with respect to the media.
We extracted the sample for the analysis from YouTube with the following parameters: the 50 most viewed videos on the UK, Italian, and Spanish regional YouTube sites (".co.uk", ".it" and ".es") and with the search keywords "COVID" or "Coronavirus". We then extracted user comments for each of the 150 videos using the company’s own API, for a total of 111,808 comments (15,933 from Spain, 27,468 from Italy, and 68,407 from the UK). Along with the comments, we downloaded other variables such as the number of "likes", the number of replies, the author’s identity, and the video’s identity. It is important to note that the data extraction, which took place on 29 December 2020, was based on popularity, so it does not represent the time period of the COVID-19 crisis, nor do the topics of the videos necessarily have to be similar (beyond dealing with the disease). To do otherwise would alter the results of the research by eliminating the selection made by citizens when approaching this social network. Once we acquired the corpus, we processed the text according to the standards of this type of study: the elimination of atypical symbols, blank spaces, and tokenisation, among other aspects (see, for example, Meyer et al., 2008). As the number of extracted comments is massive, we did not consider a qualitative exploratory technique, so useful in other cases, to be appropriate. We deemed it necessary to apply an automatic technique for extracting and reducing the information contained in the sample for description. In this way, we were able to reduce the thematic complexity contained in the almost 112,000 comments to a few themes or ideas present in most of them (divided by country). In this regard, there are a multitude of existing techniques, from supervised algorithms (García-Marín & Calatrava, 2018) to unsupervised algorithms (such as LDA and LSI). Since the sample includes very varied texts, supervised algorithms do not seem advisable since the training should be based on all existing topics in very short texts. Therefore, we decided to apply an unsupervised technique, namely, a latent Dirichlet allocation (LDA) algorithm. This algorithm is a natural language processing (NLP) technique grounded in exploring the relationships between a set of documents and the terms they contain (so it is frequency-based) by producing concepts related to those documents and terms. The algorithm assumes that words with close meanings will co-occur in similar texts. The result is a set of topics present in each of the documents (a good explanation of its operation and usefulness can be found in Letsche and Berry, 1997), which shows that it is a fairly well-established technique.
However, the polarisation analysis is more complicated. First, we measured the polarisation of each unit of analysis (comment) through the development of its own index. We did this because the measurement of affective polarisation, defined as partisan identification, normally employs the survey as a reference methodology (Druckman & Levendusky, 2019). However, such an approach cannot be applied to anonymised data from social networks (i.e. to texts). To achieve this, we performed a classification by means of sentiment analysis, which allowed us to rate and classify the sample.
Since the sample is multilingual, we decided to use an identical analysis for the three languages (although adapted to the languages through a specific dictionary for each of them) and not to use three different analyses that could bias the results. We selected the tool Orange3 (Demsar et al., 2013) based on Python (which uses a multilingual dictionary for more than 50 languages). However, since this type of analysis does not provide more information than a statement about the positivity or negativity of words or phrases, we chose a way to measure polarisation in detail (Serrano-Contreras et al., 2020). To do so, we operationalised the polarisation of a comment as the distance between the sentiment analysis of that text and the median of the aggregate of the sentiment analysis of all comments from the same country (Spain, Italy, or the UK) in absolute number. In this way, we obtained a log that can take any value between 0 and 200, where 0 denotes no polarisation (although there can be both positive and negative sentiments) and 200 indicates maximum polarisation. That is, we defined polarisation not as the expression of the valence (negative or positive) of an expression, but as the difference between these valences. Thus, an isolated comment would not express any kind of polarisation on its own, but it would if its context was mostly opposed. For example, a negative comment was not polarising if the average comment was equally negative (such as condemnation of a criminal act). However, in the same environment, a positive comment would be considered polarising if it were outside the average.
Although the composition of the index may seem synthetic, the results provided are consistent with the perceived reality, as seen below. Naturally, the index is flexible enough to allow for changes in its composition; thus, we also extracted the polarisation per video or per author. Since the sample is very large, on the order of tens of thousands of comments, the polarisation will tend toward zero, as there will be many elements that will be cancelled or directly neutral. Therefore, small changes in the number will be quite significant.
Finally, the polarisation index has served as the basis for statistical analyses to model its dependence and to expose possible differences between these countries, which is the basis of our hypothesis.
Analysis and findings
Table 1, which summarises the sample captured from YouTube, serves as an initial source for our research proposal. From the angle of frequency analysis, we can see that the videos for Spain registered the fewest comments, slightly more than half of the comments for the Italian case, and less than a quarter of the comments for the British case. This could be a consequence of lower population figures, although other factors may also be at play. Beyond these issues, the sample size is sufficiently large to consider the results of the statistical analysis meaningful.

The rest of the variables included as possible explanatory factors follow a similar pattern: Spanish is the language with the fewest authors of comments and the fewest "likes". However, some differences can be perceived in the distribution of cases. On the one hand, the authors of comments in Spanish were less active (with an average of 2.18 comments) than those in English and Italian (with respective values of 2.20 and 2.87 comments). On the other hand, comments on videos about Spain also received fewer average "likes" (3.08) than those about Italy (4.69) and the UK (9.33). Naturally, this last fact is determined by the number of authors in each language.
If we go beyond a descriptive analysis, we can note relevant findings in relation to the content of the comments. As stated above, it is difficult to describe such a large sample. For this reason, we decided to use an unsupervised technique, LDA. We found of particular interest that after this process, we identified substantial differences between the three sets of text. Table 2 depicts the results of applying the algorithm.
In all three cases, the clusters of the algorithm show a rather pejorative use of language, although with substantial differences according to the language. For example, in the Spanish case, the first group is mostly composed of insults, but not in Italian or English, where words connected with public services ("scuola", "terapia") or government ("government") are found. Derogatory language is also present in the English and Italian samples, as are references to institutions in the Spanish sample, but not in the same order (which is hierarchical according to its importance in the sample) nor in the same quantity. Allusions to government policy are also frequent, but much more so in the Spanish and English comments, but not in the Italian comments. Another interesting element may be the appearance of conspiracy and denialist elements in the English ("Liverpool", "fake", "conspiracy") and Italian (“negazionisti”, negationists) samples, but not in the Spanish sample.
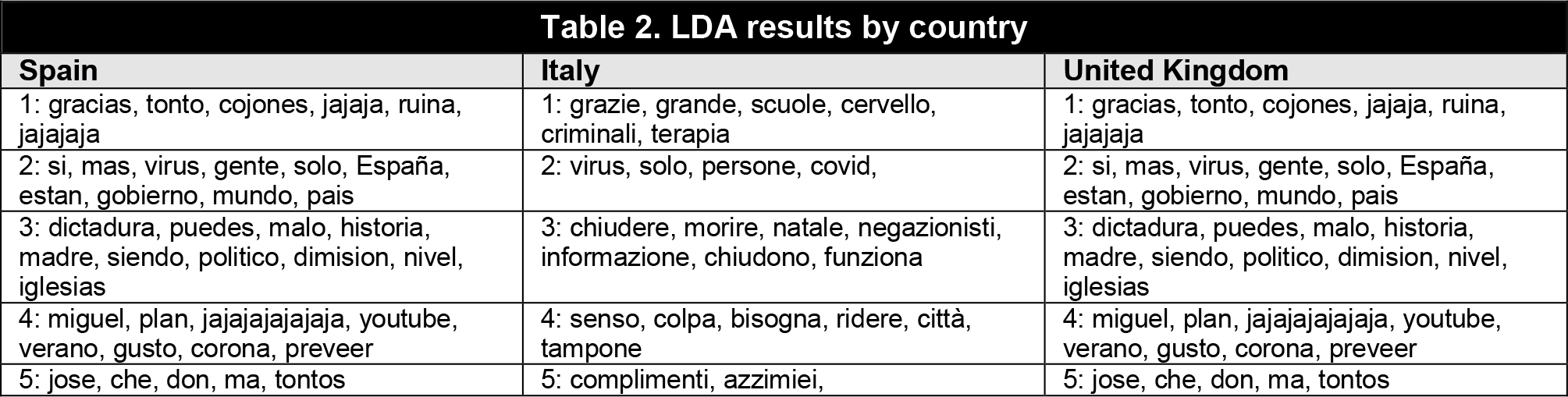
For the description of the polarisation variable, we decided to group the sample according to the language of the comments and the video or author. In other words, we measured the average polarisation of the videos and the authors of the comments, as seen in Table 3.

In the first case, the differences between each of the languages analysed are easily observable: the average polarisation is much higher in Spanish (6.91) and Italian (6.24) than in English (2.57). It is also interesting to note that the standard deviation is much more pronounced for Spanish and Italian (1.50 and 1.32, respectively) than for English (0.37). In all cases, the average polarisation may be too low (after all, the index would theoretically move from 0 to 200). However, we should remember that this is a very large sample composed, for the most part, of non-computable terms (prepositions, articles, and emojis). This means that when the whole dataset is aggregated, even by language, it tends to zero. Similarly, we can state that even if we obtain quite small differences, they are still visible.
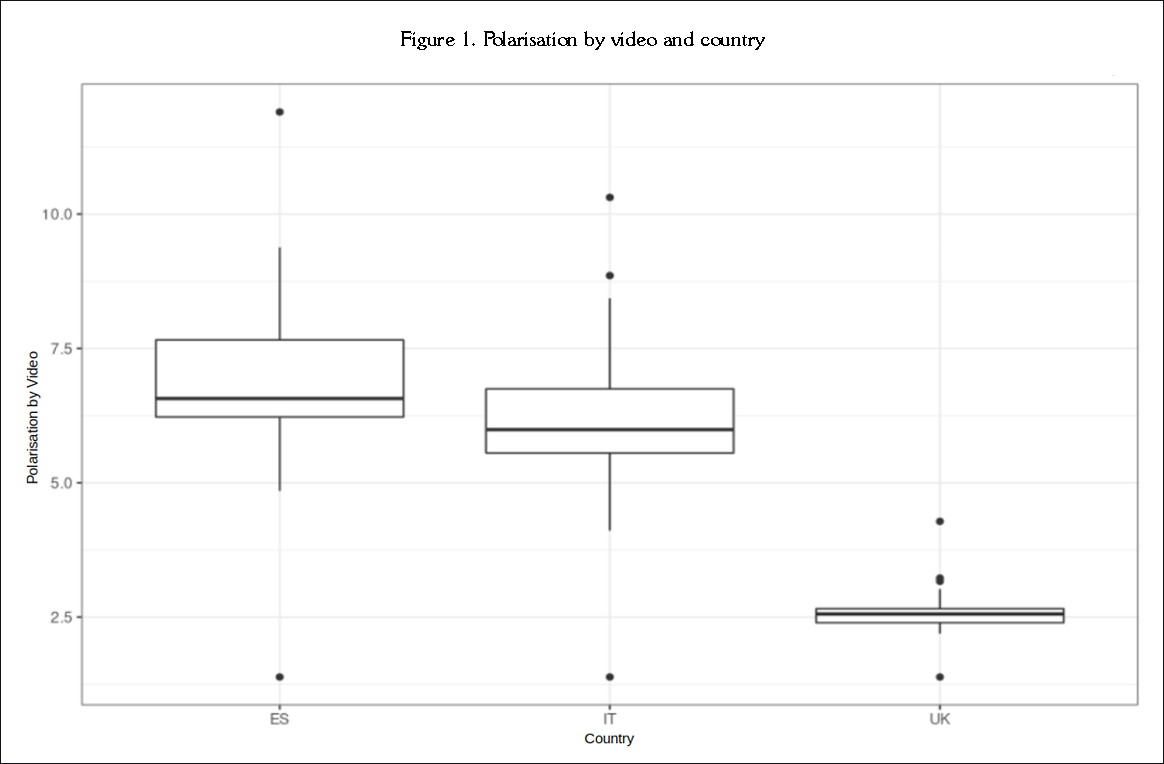
Figure 1 is a much more illustrative representation of the above. It is very clear that two different datasets are obtained: The Spanish and Italian comments, on the one hand, and the English case, on the other. Not only is the grouping of the segmented averages by case (country) striking, but also the wide dispersion for the first two cases: Spain and Italy.
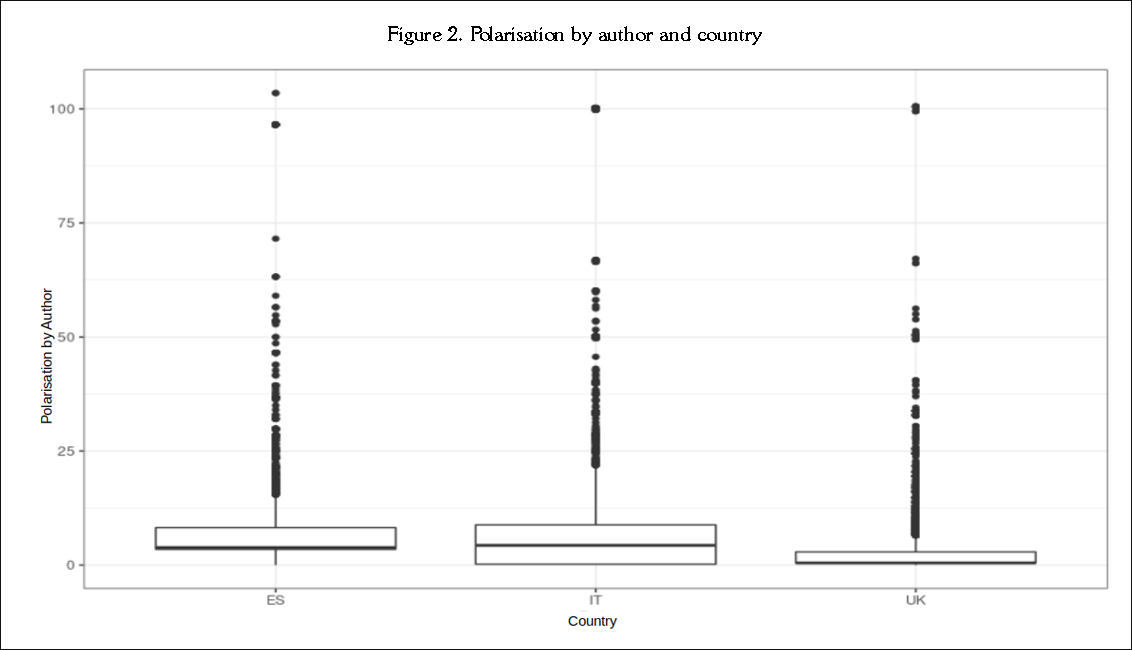
In the analysis of the comments disaggregated by author, the results reveal a similar pattern, as shown in Table 3: The average polarisation per author is highest in the comments in Spanish, followed by the comments in Italian and, at a great distance, the comments in English. It is equally interesting to see how the dispersion behaves in a different way: the minima and maxima in this case are very similar (from 0 to 100). This may mean that neither sample is free of highly polarising authors ("trolls", we could say), although these would be less numerous in the English case than in the Spanish and Italian cases. Again, Figure 2 portrays the results more clearly than the data included in the tables: although the English average is still lower than the Spanish and Italian averages, there are a multitude of equally polarising authors, but they tend to concentrate in the lower part of the graph.
Naturally, more complex statistical tests reflect a significant difference 2 between the variables "country" and "polarisation" (F=3.521, gl=2; p<0.05). The Tamhane, T3 Dunnett, and Games-Howell tests 3 indicate significant differences between the three countries and polarisation, although they are stronger between the UK-Spain sample (-4.22) and Italy (-3.54) than between the latter two (-0.67 Italy-Spain) 4 . The same happens if we add polarisation by video (F=198.4, gl=2, p<0.05; in this case, UK-ES -4.33, UK-IT -3.66 and IT-ES -0.67) or by the author of the comments (F=1.969, gl=2; p<0.05; UK-ES -5.10, UK-IT -4.40 and IT-ES -0.70).
Likewise, when crossing the variables "number of likes" and "country" in a univariate linear model, we found significant differences that explained a large part of the variability in polarisation (country*likes F=9.259, gl=6; p<0.05, R2=0.60). These results are extremely interesting because they show a different pattern for the three countries, explaining the behaviour of polarisation in the sample. In this way, we can see a divergence between the behaviour of people who commented on the British videos and those who commented on the Italian or Spanish videos, as with the data presented above. As seen in Figure 3, in the British case, the number of ‘likes’ decreases along with the polarisation. The Italian case shows a completely opposite behaviour, with the most polarising comments having the most “likes”. The Spanish case is similar to the Italian case, with the divergence that the most supportive comments are somewhat less polarising than the immediately preceding ones, but not by much (in any case, they are still more polarising than any comment in English or Italian).
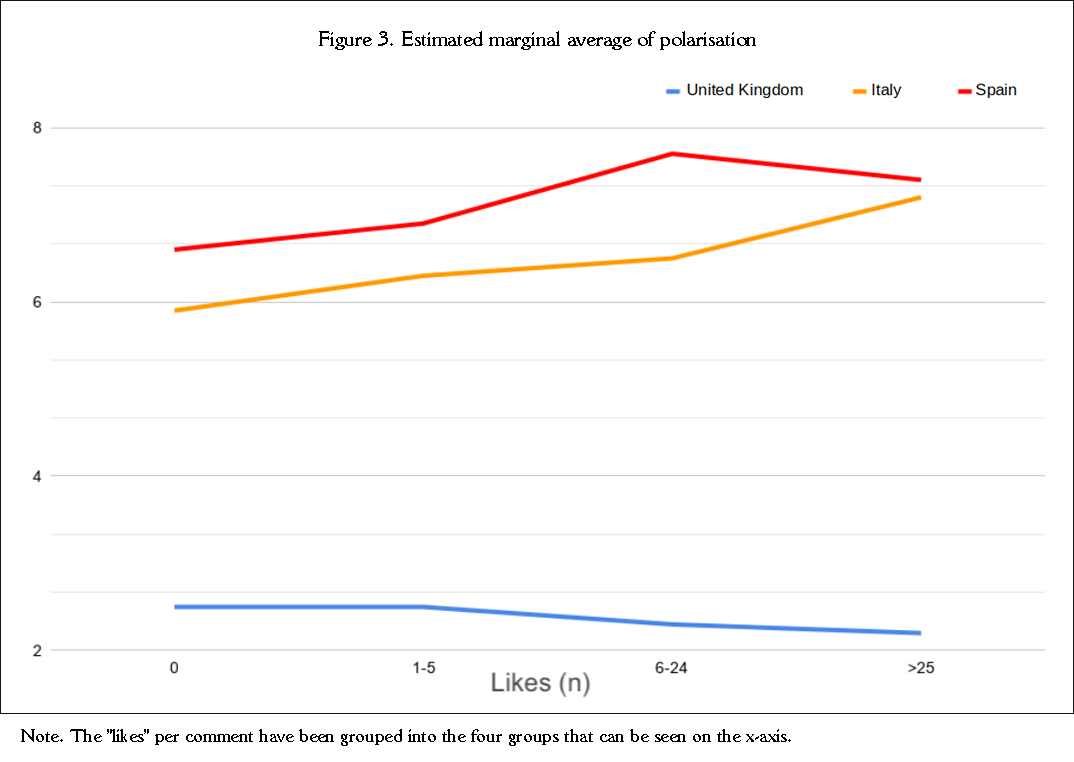
It is important to note that "likes" always follow comments, so causal relationships could be established. Although the model is far from explaining the entire sample, we consider it very significant that in each segment (language), comments are rewarded or punished according to their polarisation. In this line, we assert that cultural—rather than situational—patterns could explain the differences. Hence, for the studied sample, the variable "country" (language) is a good predictor of polarisation, and depending on it, the number of "likes" may also be a good variable to explain polarisation, either inversely (UK) or directly (Italy especially, but also Spain). However, we did not detect any significant relationships between the number of comments and polarisation by video or author or between the number of authors and polarisation, beyond the merely descriptive ones, as the sample is overrepresented in favour of comments in British videos.
Discussion and conclusions
Research on how deliberation takes place in the digital sphere has firmly established itself as a priority in disciplines such as political science, communication sciences, sociology and even computer science. We propose an innovative methodology to analyse political deliberation on social networks (YouTube) based on the use of algorithms to reach a standardised measure of polarisation.
After scrutinising the findings, we can confirm that the hypothesis underlying this proposal is verified. In countries with polarised pluralism models (Spain and Italy), treated as an independent variable, we observed a greater polarisation in political deliberation in the digital sphere in comparison with countries with the liberal model (United Kingdom). Similarly, the evidence shown specifies that in southern European countries polarising behaviour in the digital sphere is rewarded, which is not only not the case in the UK, but the opposite is true. Following Hallin and Mancini (2004), the first group of countries is characterised, among other things, by so-called political parallelism (political militancy of the media). Therefore, we interpreted this finding as a consequence of the extrapolation of the behaviour of traditional media to the digital communicative spaces that are formed in social networks. There would therefore be a process of feedback that would also infect the communicative dynamics in the digital sphere, which could be consolidated as a defining, confirmatory characteristic of the profiles that the aforementioned models propose for the political and media systems, functioning as an element of extension of the political parallelism, which is highly influenced by the political and media culture of each of the systems and is more intense in the models of southern Europe.
This happens, moreover, with an issue that can be considered commonplace, where a priori less polarisation is expected, although the role of some very active actors in the networks, such as the so-called negationists, make this a very interesting topic for discussion.
One of the most striking results of this research may help to elucidate the differences between theoretical and empirical studies on polarisation and social networks. Thus, analyses such as those by Spohr (2017), Parisier (2011), or Sunstein (2018) seem to indicate that polarisation could be a systemic effect of the functioning of information on the Internet and, more specifically, of social networks. However, empirical analyses have questioned these outcomes (Bakhsy et al., 2015; Dougan & Smith, 2016; Boxell et al., 2017; Allcott & Gentzkow, 2017). Our analysis may contribute some information to this debate by finding regional differences in the behaviour of affective polarisation in social networks, although it would not be the first contribution in this sense: Lee et al. (2014) already pointed out that polarisation could be linked to social networks but not with respect to all topics. Something similar is shown by Serrano-Contreras et al. (2020) when analysing polarisation with respect to three very different topics (elections in Spain, Catalan independence, and climate change). Above all, it seems to support the thesis of Boxell et al. (2020), who found very different behaviour across countries when examining affective polarisation from 1980 to 2015 in nine advanced democracies.
The dynamics of polarisation, described above, lead to a process of progressive fragmentation of the digital public sphere. The rupture of its unity is hence not replaced by a plural segmentation of interconnected public spheres (which seemed to occur in the initial phase of social networks, between 1997 and 2002), but by public spaces that often do not have reciprocal links or, in the best of cases, with weak links, and always under the condition of a strong polarisation process. The fragmentation of the public sphere, accelerated by digital communication ecosystems, produces a pulverisation of experiences and facilitates the emergence of resonance chambers, where the orientation action operated by algorithms does not play a secondary role in the information mechanisms. Our findings are in line with the results of previous research, pointing to the growth of filter bubbles, which play a role of ideological legitimation of social networks themselves and, more generally, of what has recently been defined as the ‘platform society’ (van-Dijck et al., 2018), and to the processes of depoliticisation, from the post-representative tendencies of Western democracies, exacerbated by social networks, to the substantial transformation of the public sphere (Schlesinger, 2020; Sorice, 2020). Further, social networks help to form islands of information that constitute a sounding board for generating communicational linkage and saturation (Morlino & Sorice, 2021).
The results of this research raise additional questions, which could be a vein for future research. On the one hand, more countries could be included in the analysis to check whether this connection between polarisation and fragmentation is also recognisable in other countries with different political-institutional systems. On the other hand, the incorporation of other platforms, such as Twitter, Facebook or Instagram, into this same logic of analysis could verify whether the structure of polarisation occurs in the same way as on YouTube. In this line, checking the behaviour of deliberation on other topics would be an interesting anchor for comparison, and would contribute to a more precise discussion of this object of study. Finally, the inclusion of controls for other aspects (such as the structure of audiences in the different countries, which could function as intervening variables) could also represent a future advancement.
Author Contribution
Idea, O.L., J.G.M.; Literature review (state of the art), O.L., J.G.M., E.D.B.; Methodology, O.L., J.G.M.; Data analysis, O.L., J.G.M.; Results, O.L., J.G.M.; Discussion and conclusions, O.L., J.G.M.; Writing (original draft), O.L., J.G.M.; Final revisions, O.L., J.G.M., E.D.B.; Project design and sponsorship, O.L., J.G.M., E.D.B. (1)