Citizen participation in Twitter: Anti-vaccine controversies in times of COVID-19
Abstract
Twitter has transformed into one of the main platforms for citizen engagement today. However, even though previous studies have focused on opinions about vaccines in general or about specific vaccines, opinions towards COVID-19 vaccines on Twitter have not been researched to date. The objective of this research is, by using social network analysis and language processing tools, to examine the degree to which the opinions and interactions present on Twitter are favorable or unfavorable towards the main COVID-19 vaccines. In addition, the relevance of each of the vaccines is studied, as well as their level of controversy. Likewise, the present study investigates, for the first time, the conversation from different perspectives including the content and also the participants, by analyzing in detail the verified accounts and using tools for the detection of bots. In global terms, the results from verified accounts show a moderate favorability towards the COVID-19 vaccines, the most accepted being those of Oxford-AstraZeneca, Pfizer, Moderna, and Sputnik V. On the other hand, the vaccine that attracts the most attention is the Russian Sputnik V, which is also the most controversial, behind those developed in China. Finally, verified users are shown to be relevant agents in the conversation due to their greater capacity for dissemination and reach, while the presence of bots is practically non-existent.
Keywords
Network analysis, quantitative analysis, misinformation, virtual communities, social media, critical thinking
Palabras clave
Análisis de redes, análisis cuantitativo, desinformación, comunidades virtuales, redes sociales, pensamiento crítico
Resumen
Twitter se ha transformado en una de las principales plataformas de participación ciudadana hoy en día. Sin embargo, aun cuando estudios similares previos se han centrado en la opinión sobre las vacunas en general o sobre otras vacunas específicas, hasta la fecha no se han investigado las opiniones hacia las vacunas contra la COVID-19 en Twitter. El objetivo de esta investigación es, mediante el uso de herramientas de análisis de redes sociales y de herramientas de procesamiento del lenguaje, examinar el grado en el que las opiniones e interacciones presentes en Twitter son favorables o no hacia las principales vacunas de la COVID-19. Además, se estudia la relevancia de cada una de las principales vacunas, así como su nivel de controversia (polemicidad). Igualmente, el presente estudio investiga por primera vez la conversación no solo desde el punto de vista del contenido, sino también de los participantes que la integran, analizando en detalle las cuentas verificadas y empleando herramientas para la detección de bots. En términos globales, los resultados muestran una moderada favorabilidad hacia las vacunas de la COVID-19, siendo las más aceptadas las de Oxford-AstraZeneca, Pfizer y Moderna, y la de Sputnik V en el caso concreto de las cuentas verificadas. Por otro lado, la vacuna que más atención acapara es la rusa Sputnik V, que es además la más polémica por detrás de las de origen chino. Por último, los usuarios verificados se muestran como agentes relevantes de la conversación por su mayor capacidad de difusión y alcance, mientras que la presencia de bots es prácticamente inexistente.
Keywords
Network analysis, quantitative analysis, misinformation, virtual communities, social media, critical thinking
Palabras clave
Análisis de redes, análisis cuantitativo, desinformación, comunidades virtuales, redes sociales, pensamiento crítico
Introduction and background
On March 11, 2020, the World Health Organization (WHO) attributed global pandemic status to COVID-19 in light of the alarming levels of disease transmission (World Health Organization, 2020a). Since then, the economic, social, and health consequences of COVID-19 have been, and continue to be, profound (United Nations, 2020; Yelin et al., 2020).
In a context in which the main containment strategies employed in most countries (social distance, confinement, restrictions on tourism, and other services...) have proven to be extremely costly from an economic point of view and insufficient to halt the advance of the virus, the main hopes for ending the pandemic lie in the development of specific drugs and, especially, vaccines (World Health Organization, 2020b). However, despite their proven efficacy in saving lives, as well as containing and eradicating diseases (Andre et al., 2008), vaccines have often been surrounded by controversy (Dubé et al., 2015) and have even suffered, in many cases, from direct opposition (Hornsey et al., 2018).
The reasons for this mistrust derive, among other reasons, from a postmodern cultural context that questions the legitimacy of science, the pharmaceutical industry, and medical authority (Bertin et al., 2020), and from a set of controversies about possible negative side effects associated with vaccines (e.g., Spier, 2001). Although studies have found no evidence to support such assumptions (Flaherty, 2011; François et al., 2005), doubt and suspicion have spread across the globe (Poland & Spier, 2010). All this has led the WHO to include distrust of vaccines in the list of the top ten global health threats (Friedrich, 2019).
In this regard, the COVID-19 vaccine is no exception, and one of the main reasons for distrust among those who speak out against receiving the vaccine is the fear of possible side effects, which is far ahead of other possible reasons, including the speed with which clinical trials have been conducted (Dror et al., 2020; World Economic Forum, 2021).
Much of this dialogue about the pandemic and the appropriateness of vaccines has moved from conventional media to social networks (Schmidt et al., 2018; Cuesta-Cambra et al., 2019) although not always with guarantees of reliability in the face of fake news (López-Rico et al., 2020). The debate is especially intense on Twitter (Himelboim et al., 2020; Meyer et al., 2019; Puri et al., 2020), which has become one of the main tools for channelling citizen participation (Auger, 2013). Among all social communication platforms, Twitter is unique in its ability to share and disseminate ideas in a fast and barrier-free manner due to its unique characteristics. For example, unless intentionally chosen otherwise, all tweets are immediately visible to everyone by default. In addition, it has mechanisms such as hashtags that facilitate dialogue on specific topics without the need to create delimited groups. Further, its retweet function makes it possible to disseminate information easily and immediately. Finally, unlike other networks such as Facebook, Twitter does not require reciprocal relationships between users which favours the emergence of central nodes for information dissemination (Colleoni et al., 2014).
As such, Twitter has become the worldwide platform that collects the opinions and information exchange of citizens regarding political (Manfredi-Sánchez et al., 2021), environmental (Vu et al., 2020), and social issues such as immigration (Gintova, 2019) and gender equality (Puente et al., 2021). Likewise, citizens have begun to use Twitter as a forum from which to claim civil rights (Yang et al., 2017), as a platform to denounce the violation of such rights (Xiong et al., 2019), and to carry out activist campaigns (Bosch, 2017).
Within the health domain, previous literature has analysed the debate that has arisen on Twitter about different specific vaccines and vaccines in general (Bello-Orgaz et al., 2017, Broniatowski et al., 2018; Himelboim et al., 2020; Milani et al., 2020; Tomeny et al., 2017). However, due to its recent nature, there has been no opportunity to analyse citizen dialogue regarding COVID-19 vaccines. Studying and monitoring the opinions and information shared on Twitter around a socially relevant topic such as vaccines against this coronavirus is important, first, because it can help the scientific-medical community to identify concerns, interpretations, and misconceptions, and to design specific messages that serve to clarify or disprove them. Secondly, because such opinions can be representative of the social climate of opinion in its entirety (Graells-Garrido et al., 2019); this allows predictions to be made regarding the subsequent behaviour of citizens (Burnap et al., 2016). Thirdly, the study of such opinions and content is relevant because, beyond its representativeness, it can be a key factor in shaping or influencing the attitudes of the participants of the conversation being able to become, in this particularly sensitive area, a tool of information or disinformation that propagates unscientific theories and opinions that have real health, economic, and social consequences for the population as a whole (Kouzy et al., 2020) since an increasing number of people are turning to the Internet for information on whether or not to be vaccinated (Jolley & Douglas, 2014).
On the other hand, to date, most previous research on platforms such as Twitter does not differentiate between different audiences when analysing discussions and opinions. However, given that this network allows for the identity and specific data of its users (such as their professional careers, for example) to be recorded through verified accounts (i.e., those accounts identified by Twitter with a blue badge which confirm their authenticity), it is relevant to study the extent to which opinions on a medical and scientific topic such as these vaccines vary according to these characteristics. This approach is novel since, with some exceptions (Sued-Palmeiro & Cebral-Loureda, 2020), practically all research on Twitter has focused mainly on studying the content of messages, but with little attention paid to the individuals who generate and disseminate them. Specifically, identifying the presence and behaviour of different social sectors can be of great use to health professionals and institutions as it would allow not only to detect currents of opinion and specific needs but also to design and disseminate specific interventions for certain sectors (Tomeny et al., 2017).
At the same time, taking a closer look at the behaviour of those users who have verified accounts brings an additional advantage since the users of such accounts have differential qualities and requirements. Specifically, only those accounts that are qualified by Twitter as being of "public interest" can be verified, i.e., the account must belong to a socially relevant issuer and be active (Twitter, 2021). These accounts, therefore, are of special interest since, by their very definition, they can exert greater influence on the conversation inside and outside the platform.
Moreover, its use not only allows us to identify personal information that facilitates the social categorization of individuals participating in the dialogue, but also to avoid the presence of malicious agents such as bots, trolls, etc., that distort and adulterate the conversation (Subrahmanian et al., 2016). As such, previous research has already highlighted the existence of bots in vaccine debates where they play the role of disseminators of anti-vaccine messages (Broniatowski et al., 2018). Therefore, it is also of interest to shed light on their presence and role in the conversation about the COVID-19 vaccine currently taking place on Twitter.
In short, the present study is guided by the following research questions:
-
Which are the most relevant vaccines? Which vaccine generates the most participation and dialogue?
-
What is the sentiment or favourability towards the main COVID-19 vaccines?
-
What is the specific activity carried out by the verified accounts? Are there differences in favourability of the different vaccines based on the different professional categories of these users?
-
How often are bots present in the conversation? What is their activity?
Methods and materials
In order to study Twitter conversations, Social Network Analysis methodology (SNA) was used (Brand & Gómez, 2006). In the first phase, tweets related to the object of study were downloaded using NodeXL pro (Hansen et al., 2010), a program for obtaining social network data, and then processed through SNA. To obtain the data, all the tweets published and their respective interactions (replies, mentions, retweets, and mentions in retweets) that cited any vaccines that were in Phase 3 of their research were downloaded, these vaccines include, Moderna, Pfizer, three vaccines of Chinese origin (Cansino, Sinovac and Sinopharm), Sputnik V, and Oxford-AstraZeneca.
To select the days of greatest interest concerning vaccine discussions, data was obtained during the first four days after efficacy data were announced for each of the four vaccines that had made their results public before November 30, 2020 (Pfizer, November 9; Sputnik V, November 11; Moderna, November 16; Oxford-AstraZeneca, November 23) (AJMC, 2020; Callaway, 2020). On each of these four days, tweets were downloaded that also alluded to, in addition to the four vaccines mentioned above, three others that were in Phase 3 (the Chinese CanSino, Simovac, and Sinopharm). The program downloaded a total of 49,776 interactions by 25,692 Twitter users, of which 2,970 were original tweets. Sometimes tweets referred to more than one vaccine, so after the number of tweets mentioning each vaccine was counted, those messages mentioning several vaccines were counted once for each vaccine mentioned. Additionally, the data collection tool did not count favourites as an interaction, so these favourites were later counted separately.
Study variables
Relevance: To identify the relevance of each vaccine, the number of users was counted as well as the number of tweets that mentioned each vaccine. Different indices were then generated to represent different relevance parameters:
-
Total Activity Index is the proportion in which the users of each vaccine conversation participated in the conversation (no. of interactions/no. of users). This index makes it possible to determine which of the vaccines had the most activity on the network.
-
Original Activity Index is the proportion of users in the conversation for each vaccine who participated in the conversation by issuing an original tweet (no. of tweets/no. of users). This index helps determine whether there was a greater initiative on the part of the users to start a discussion through original tweets.
-
Reaction Index is the degree to which tweets about a particular vaccine were able to provoke interactions by the recipients (no. interactions/no. tweets in which the vaccine is mentioned).
Favourability: To establish the degree to which users were favourable to COVID-19 vaccines in general, as well as to each of them, a "Favourability Index" was created. This index is presented as the sum of those reactions that indicate acceptance or agreement with the content of a tweet (retweets and favourites) multiplied by the sentiment or polarity (either positive or negative) of that tweet. Higher values in this index reflect greater support for each of the vaccines, while lower values will imply greater rejection.
To calculate the sentiment or polarity index of each tweet, the TextBlob Sentiment Analysis tool (Loria, 2020) was used through Python (Oliphant, 2007). TextBlob is a library programmed in Python for processing textual data. It provides an API to perform natural language processing (NLP) tasks, such as part-of-speech tagging, noun phrase extraction, sentiment analysis, classification, and translation among other utilities. In this case, the polarity returned by the tool using a lexicon method for each tweet is a numerical value within the range [-1.0, 1.0] where -1 identifies a message with a very negative sentiment and 1 with a very positive one. The level of effectiveness of the program has been previously contrasted in published research (Fauziyyah, 2020; Micu et al., 2017).
Controversy: To establish the degree to which the conversation is or is not controversial (i.e., includes both favourable and unfavourable opinions), a "Polemic Index" was created (see Denia (2020) for a conceptually similar index). This index aims to capture the ratio between the number of reactions provoked by tweets mentioning a vaccine and the favourability of those reactions (reaction index/favourability index). Higher values in this index suggest a higher degree of controversy.
Verified accounts and professional sectors: The tweets that came from users whose accounts were verified by Twitter were identified and examined, as this was considered to be the only way to ensure that the person behind the user account was truly the one indicated. Once these users (973) were identified, they were grouped into the 16 most repeated categories, as well as an "other" category. These groups were then sorted into four major categories or sectors with their corresponding subcategories, as follows: Science and health (scientific journals, scientific associations, scientists, healthcare, and pharmaceuticals); Communicators and media (journalists, media, communicators); Politics and government (politicians and public institutions and administrations) and Civil society (athletes, lawyers, artists, economists, sports institutions, and others).
Diffusion: To measure the degree of diffusion of each user's messages. This index was established by multiplying the number of followers of each user by the number of retweets of their messages. Higher values indicate greater reach or viral capacity of the user.
Bots: To identify the degree to which bots were present in the conversation as well as their most common behaviours, the Python library, Botometer, (Botometer, 2020; Yang et al., 2017), was used. This detection system, which has been commonly used for the identification of bots in Twitter conversations (e.g., Broniatowski et al., 2018), analyses a user’s characteristics to different variables and returns a value in an interval between 0 and 1 where 1 indicates that a user’s characteristics match those of a bot 100%, and 0 when they do not. A user with a value of 0.3, for example, indicates that a user has the same characteristics as a bot 30% of the time. When analysing the differences between variables, statistical tests were used to show whether these differences were statistically significant. If the data did not show a normal distribution, non-parametric tests such as Kruskal-Wallis (Ostertagova et al., 2014), Mann-Whitney U (McKnight & Najab, 2010), or Chi-square (McKnight & Najab, 2010) were used.
Analysis and results
Relevance of vaccines in the network
As can be seen in the data collected in Table 1, the most relevant vaccine in terms of the number of users who talked about it, as well as the number of tweets that were published mentioning it, was the Russian vaccine (Sputnik V), followed by Pfizer, Moderna, Oxford-AstraZeneca. and those manufactured in China. Table 2 data shows that the vaccines with the most active interlocutors were Sputnik V (each user averaged 7.73 interactions), followed by Pfizer (5.39), Moderna (4.74), Oxford (4.37), and the Chinese vaccines (2.74). Similarly, the Russian vaccine provoked the most reactions (72.56 interactions per tweet posted), ahead of Oxford-AstraZeneca (66.73), Pfizer (61.41), Moderna (60.48), and the Chinese-produced vaccines (21.32).
Favourability towards vaccines in conversation
The polarity analysis tool used indicated that the average overall sentiment towards all COVID-19 vaccines was moderately favourable or positive (M=0.11; SD=0.19). When polarity or sentiment towards each vaccine was analysed, the results showed that polarity values could be grouped into three levels with Pfizer (M=0.16; SD=0.198) and Moderna (M=0.16; SD=0.19) as the vaccines with the highest positive values. A second group was found within the set of three vaccines of Chinese origin (M=0.13; SD=0.17) and the Oxford vaccine (M=0.12; SD=0.18) with lower mean relative positive sentiment values for the Russian Sputnik V vaccine (M=0.098; SD=0.19). The analysis revealed that these differences were statistically significant (Kruskal-Wallis (4)=53.022; p<0.001). On the other hand, when the favourability index was calculated (by taking into account not only the sentiment or polarity of each tweet, but also the number of favourites and retweets) for each of the vaccines (Table 2), the results showed that the Oxford-AstraZeneca vaccine received by far the highest acceptance (M=21.58; SD=54.70), followed by Pfizer (M=13.85; SD=59.25), Moderna (M=10.23; SD=25.17), Sputnik V (M=7.88; SD=58.05), and finally the three Chinese vaccines (M=1.34; SD=5.23) which, although they did not generate negative sentiment, was rather neutral. These differences between the vaccines were also identified as significant (Kruskal-Wallis (4)=70.337; p<0.001).
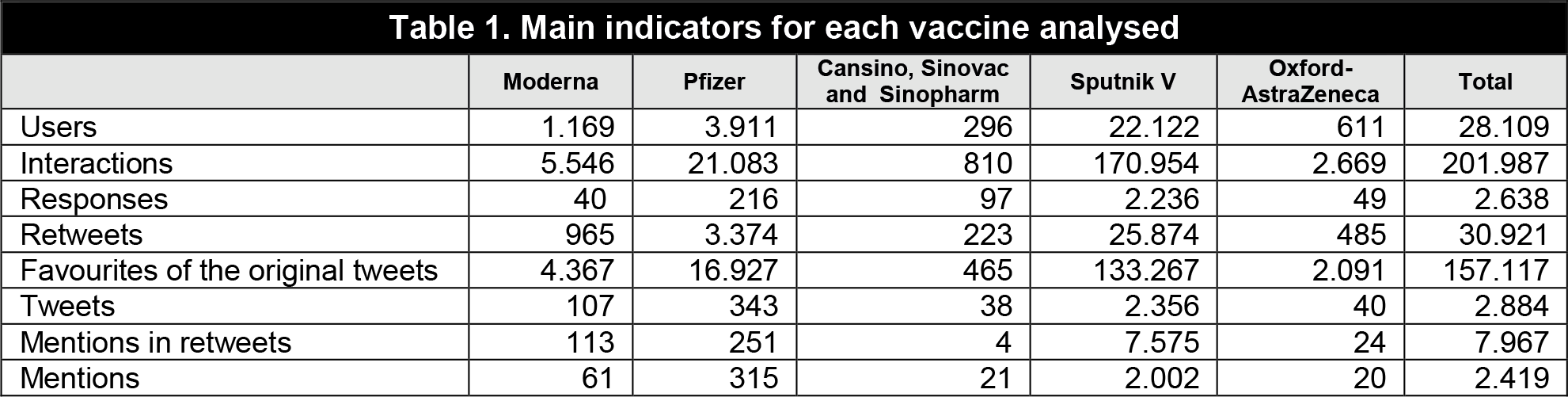
Finally, the vaccines with a significantly higher degree of controversy (Polemic Index) than the rest were the Chinese vaccines with an index of 15.82, followed by the Russian vaccine (9.21). The vaccines with the lowest level of controversy and discussion were Moderna (5.06), Pfizer (4.44), and Oxford (3.09). (Kruskal-Wallis (4)=47.370; p<0.001).
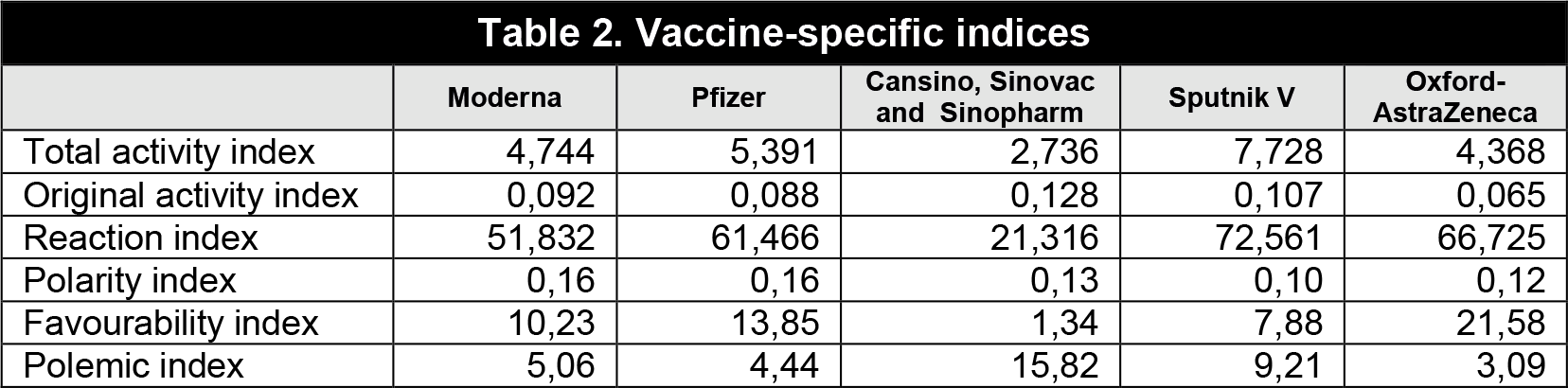
Verified accounts
Of the total number of tweets (2,868), the authors were identified and tweets were catalogued according to whether their user account was verified or not, which made it possible to identify the person or institution behind each. The data showed that 22.7% of the tweets came from users with verified accounts.
Through the analysis of the type of activity carried out by this type of user, the data indicated that, as expected, 47.11% were focused on the publication of original tweets, while mentions in retweets occupied 20.55% of their activities. This was followed by replies to original tweets (16.14%), mentions to other users (9.55%), with retweeting messages from other participants (6.66%) being their least common activity. As can be seen in Table 3, since users of verified accounts are more prolific than other participants, they showed significantly more activity compared to users in the general sample.
In turn, when the main variables (relevance, favourability, and controversy) were analysed by examining only users with verified accounts (Table 3), the results showed that for these participants, the most relevant vaccine in terms of the number of users involved in the conversation as measured by their activity and the number of reactions generated continued to be the Russian Sputnik vaccine, followed by Pfizer’s.
Regarding favourability, although Pfizer is the vaccine with the most positive polarity or sentiment index (0.19), this time it was the Russian Vaccine Sputnik V which, when considering the number of favourites and retweets, has a higher favourability index (19.71) among the verified users, although the differences were not significant (Kruskal-Wallis p>0.05).
The greatest controversy among verified users corresponds by far to the Chinese vaccines (13.18) followed by Sputnik V (4.99), Moderna (4.25), and Oxford-AstraZeneca (3.96), while Pfizer generated the least controversy (3.05). In this case, the differences were not statistically relevant (Kruskal-Wallis p>0.05).
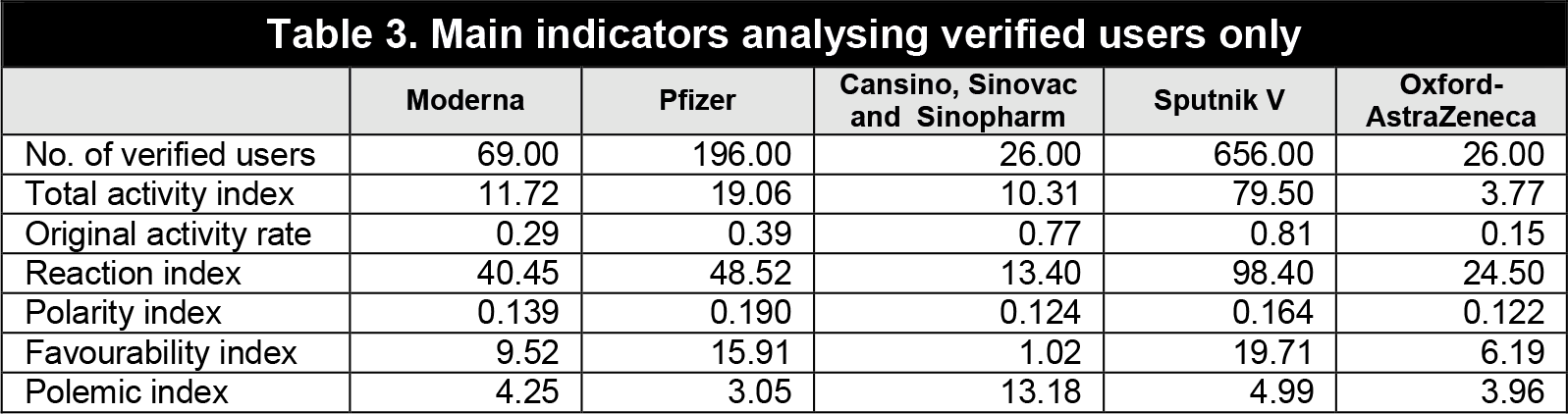
When the Mann-Whitney U test was performed to compare the sentiment or polarity of the messages according to whether the account of the author of the tweet was verified independent of any vaccine being mentioned, significant differences were found (U=848.358; p<0.001). Specifically, the mean sentiment of tweets from verified accounts was more positive (M=0.16; SD=0.20) than from unverified accounts (M=0.099; SD=0.20).
Consequently, it was relevant to analyse the diffusion of messages from verified accounts compared to unverified ones. To create this "diffusion index", the number of followers of each author’s tweet was multiplied by the number of retweets it received. When the data were analysed, the results showed relevant differences (Mann-Whitney U=1,280,576; p<0.001). The average diffusion of the verified accounts was 30,567,660.99 (SD=137,569,250.48), while the unverified ones obtained a diffusion of 635,946.47 (SD=4,757,267.29). In other words, the tweets of verified users reached more users in the network than the tweets of unverified users.
In turn, the classification of the verified accounts that had published an original tweet according to their professional sector revealed a majority of participants belonging to the category of media and communicators (95.7%). The remainder was made up of users classified as civil society (1.84%), science and health (1.38%), and politics and government (1.08%). When the differences between the categories were analysed in terms of polarity and general favourability towards vaccines, the results were not significant (Kruskal-Wallis p>.05).
Presence and activity of bots in conversation
The bot index that the analysis tool generated for each user was categorized into three groups. First, users with a value below 20% as very likely human, those with a value above 80% as likely bot, and the rest an intermediate probability. The frequencies indicated that 63.9% of the users were very probably human and only 0.3% probably bots, indicating an exceptionally low presence of the latter.
This index was not equally distributed among the different vaccines (x2(8)=21.25; p<0.01), however. The vaccines of Chinese origin presented significantly more users with a high probability of being bots (1.6%) compared to the others which ranged from 0.2% to 0.3%.
The most common activities presented by users identified as bots were those that might be expected: 58.25% of them were retweets and 13.11% are mentions with retweets. These were actions aimed at disseminating other users' messages, which is the main function of a bot. In turn, they allocate 12.14% of their actions to mentions, 8.74% to the publication of original tweets, and finally, 7.77% to replies.
Discussion and conclusions
In a context of where digital communication is gaining greater presence, Twitter has become one of the most relevant platforms for citizen participation and opinion today by hosting debates on topics as diverse as politics, the environment, social reform, and health (Auger, 2013). However, the conversation about a seemingly controversial topic as relevant from a social, economic, and health point of view as that of COVID-19 vaccines has not yet been analysed. For this reason, the present research has focused on the study of both the opinions and information expressed about the main COVID-19 vaccines and the behaviour of the participants who make up this conversation on Twitter.
The results obtained indicate that, despite the apparent media controversy, the general sentiment towards these vaccines was moderately positive. These data agree with the latest surveys conducted in 15 countries which showed a broad acceptance of COVID-19 vaccines by the majority of the population (World Economic Forum, 2021). They also coincide with Spain’s results, for example, where surveys also showed greater favourability towards vaccines, especially as their arrival was confirmed (CIS, 2021). Although we should be cautious with the interpretation of the data since they only allow us to observe an image of a specific moment, these results can be relevant because they suggest that the analysis of citizen participation collected on Twitter can have a certain diagnostic capacity about the opinions of the general population by being both a faster and less costly method than traditional surveys. Although the methodology used does not allow us to examine the specific contents of these opinions and, therefore, does not allow us to identify misconceptions or imperceptions about COVID-19 vaccines, the study of their favourability does at least allow us to infer the dominant climate of opinion on the platform which can then provide an approximate view of the general climate of opinion. As noted in the introduction, this climate of opinion is relevant because it can be used to make predictions about the subsequent behaviour of citizens which, in this particular case, could translate into a large percentage of citizens willing to receive the vaccine. In addition, the climate of opinion on the platform may also have had consequences in shaping or influencing the attitudes of the participants in the conversation. This apparent majority acceptance towards vaccines is important news as the open existence of discrepancies can suggest that there is not enough scientific consensus on vaccines, thus reducing confidence in them (Dixon & Clarke, 2013).
In turn, when studying the differences in acceptance among the different vaccines, the research found that those receiving more favourable treatment were those of Oxford-AstraZeneca, Pfizer, and Moderna, indicating a greater confidence in vaccines of Western origin than those manufactured in countries such as China and Russia whose quality standards are less transparent.
On the other hand, and for the relevance of each of the vaccines, the Russian Sputnik V was by far the one that generated the most conversation, the one with the most active interlocutors, and the one that elicited the most reactions. It should be noted, therefore, that monopolizing much of the conversation, as it is with Sputnik V, does not improve the perception of the vaccine. In fact, after the Chinese vaccines, the Russian vaccine is the one that generated the most polemic and controversy, with a high proportion of positive and negative messages. However, the present research highlights the relevance of paying attention not only to the content of the conversation but also to the nature of its participants. Specifically, this research reveals the convenience of studying in detail those users with verified accounts, since, as the data indicated, these are users who, with respect to this topic, were not only more active but also demonstrated a diffusion power almost fifty times higher than non-verified users. In addition, half of their activity is devoted to publishing original tweets, i.e., generating their own content and much less replicating what others say, which demonstrates a greater commitment to the conversation than other participants. One possible explanation of this could lie in the fact, at least as far as the conversation about vaccines is concerned, that these are users who are in the communication sector. Finally, it is interesting to note that their messages, compared to the rest of the users, were significantly more positive towards vaccines, indicating greater support and acceptance of vaccines. Therefore, our research suggests that any health authority wishing to convince the population of the proven benefits of vaccines could have an effective ally in them. Furthermore, the analyses indicate that the presence of bots, which can distort the analysis of the conversation under study, was practically marginal. However, it is interesting to note that almost all the accounts that matched the profile of a bot were related to vaccines of Chinese origin. Finally, given that our study examines only tweets published in Spanish, future research would benefit from extending the analysis to all messages written in other languages, especially English, to gain a more global perspective of the conversation. It would also be desirable for future research to examine the temporal evolution of the debate, incorporate new emerging vaccines into the analysis, and include new study variables such as the objectivity and subjectivity of the messages.
Author Contribution
Idea, R.C, M.A., E.V.; Literature Review (state of the art), M.A., E.V.; Methodology, R.C., M.A.; Data analysis, R.C.; Results, R.C.; Discussion and conclusions, M.A., E.V.; Writing (original draft), R.C., M.A., E.V.; Final revisions, R.C.; Project design and sponsorships, M.A., R.C. (1)